Francis wyffels
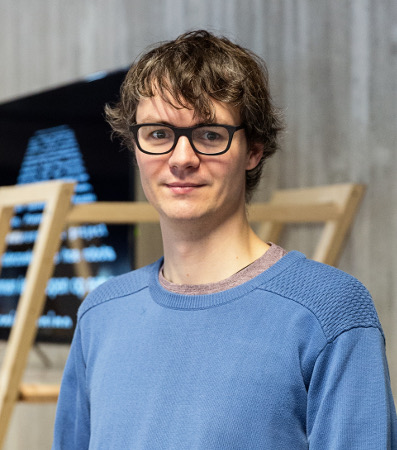
Francis wyffels
tel.: +32 9 264 95 26
research unit: Internet Technology and Data Science Lab (IDLab)
Francis wyffels is an early career professor with expertise in the domain of machine learning and robotics. In the past he has been involved as a researcher in the European FP7 AMARSi project on robot control. In the AMARSi project he focussed on training recurrent neural networks and reinforcement learning for control of robots. With his expertise in the domain of neural networks, including deep learning and deep reinforcement learning, he is well fit to coordinate the robot related work packages within this project. Francis wyffels strongly believes in dissemination activities for which he has been awarded with the UGent Hermes award for public/scientific engagement and knowledge transfer and the Google RISE award. By means of international projects with children, students and schools, he wants to ensure a more realistic perspective on what robotics can achieve, and hopefully reduce the almost innate fear for robotics. This fear is unfortunately still present in today’s society where robots are often seen as either world dominance seeking creatures or unemployment causing objects while the productivity gains and increased convenience robots bring, often remains overlooked.
Keywords: Robot control, embodied cognition, (deep) reinforcement learning, computer science education
- Urbain G., Degrave J., Carette B., Dambre J., wyffels F. (2017) Morphological Properties of Mass-Spring Networks for Optimal Locomotion Learning, Frontiers in Neurorobotics 11:16. (Locomotion control of a physical reservoir system implemented by a spring-mass-damper system)
- Vandevelde, C., wyffels, F., Vanderborght, B., & Saldien, J. (2017). DIY design for social robots. IEEE ROBOTICS & AUTOMATION MAGAZINE (in press).
- Fiers M., Van Vaerenbergh T., wyffels F., Verstraeten D., Schrauwen B., Dambre J., Bienstman P. (2014) Nanophotonic Reservoir Computing with Photonic Crystal Cavities to Generate Periodic Patterns, IEEE Transactions on Neural Networks and Learning Systems 25 (2), pp. 344–355. (Generating periodic patterns with a reservoir system with output feedback implemented by photonic crystal cavities)
- wyffels F., Li J., Waegeman T., Schrauwen B., Jaeger H. (2014) Frequency modulation of large oscillatory neural networks Biological Cybernetics 108(2), pp. 145–157. (Generating stable frequency modulatable patterns with reservoir computing systems with output feedback)
- Waegeman T., wyffels F., Schrauwen B. (2012) Feedback Control by Online Learning an Inverse Model, IEEE Transactions on neural networks and learning systems 23:10, pp. 1637–1648. (Control applications with reservoir systems with output feedback)