Steven Verstockt
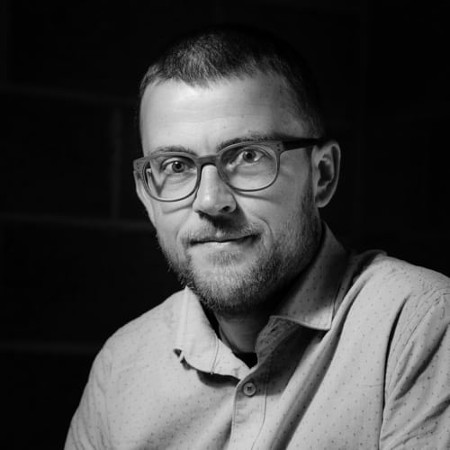
Steven Verstockt
tel.: +32 9 331 49 48
research unit: Internet Technology and Data Science Lab (IDLab)
Prof. dr. Steven Verstockt received his master’s degree in informatics from Ghent University (Belgium) in 2003. In 2008, he began working on his Ph.D. degree on multi-modal video fire analysis at the Multimedia Lab of the Electronics and Information Systems Department of Ghent University–iMinds, where he has worked since 2012 as a postdoctoral researcher. In September 2015 he started a tenure track professorship in Multimedia. His current research focuses on multimodal data processing, video analysis, computer vision and GEO-ICT. Over the last years, his research group is getting involved in several sports, media and heritage-related projects/proposals to share their expertise on spatio-temporal data collection, filtering, classification, enrichment, mapping and visualization.
Keywords: spatio-temporal (meta)data enrichment, computer vision, geo-ict, multimodal sensing, sports data science
- Geolocalization of crowdsources images for 3D modeling of city points of interest. Verstockt, S., Gerke, M., and Kerle, N. (2015). In: IEEE Geoscience and remote sensing letters, Volume: 12 , Issue: 8, Page(s): 1670 – 1674
- Verstockt, Steven, Viktor Slavkovikj, Pieterjan De Potter, and Rik Van de Walle. 2014. “Collaborative Bike Sensing for Automatic Geographic Enrichment.” Ieee Signal Processing Magazine 31 (5): 101–111.
- Fireground location understanding by semantic linking of visual objects and building information models, Florian Vandecasteele UGent, Bart Merci UGent and Steven Verstockt UGent (2017) FIRE SAFETY JOURNAL. 91. p.1026-1034
- Unsupervised spectral sub-feature learning for hyperspectral image classification. Viktor Slavkovikj UGent, Steven Verstockt UGent, Wesley De Neve, Sofie Van Hoecke UGent and Rik Van de Walle UGent (2016) INTERNATIONAL JOURNAL OF REMOTE SENSING. 37(2). p.309-326.
- Spott: On-the-Spot e-Commerce for Television Using Deep Learning-Based Video Analysis Techniques. ACM Transactions on Multimedia Computing, Communications, and Applications (TOMM) - Special Section on Deep Learning for Mobile Multimedia. Volume 13 Issue 3s, August 2017. Article No. 38