Sven Degroeve
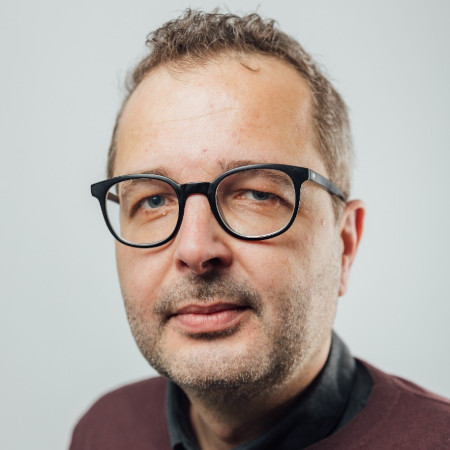
Sven Degroeve
tel.: +32 9 224 98 54
research unit: VIB Center for Medical Biotechnology (VIB CMB)
Sven Degroeve is an associate professor in Machine Learning methods for biomedical data analysis at the Department of Biomolecular Medicine of the Faculty of Medicine and Health Sciences of Ghent University. He is a staff scientist at the VIB-UGent Center for Medical Biotechnology of VIB, both in Ghent, Belgium. With over two decades of experience in Machine Learning methods, he has applied this knowledge to the fields of Natural Language Processing, Genomics, and Proteomics. Currently, his team’s research focuses on enhancing high-throughput Proteomics data analysis through the implementation of deep learning models that simulate molecule behavior across various biotechnology platforms. His work has been published in high-impact journals and has received a total of 8000 citations.
Keywords: Machine Learning, Deep Learning, Bioinformatics, Biomedical Data Analysis, Protein modeling, High-throughput omics
- Palmblad, M., Böcker, S., Degroeve, S., Kohlbacher, O., Käll, L., Noble, W. S. & Wilhelm, M. Interpretation of the DOME Recommendations for Machine Learning in Proteomics and Metabolomics. J. Proteome Res. (2022).
- Bouwmeester, R., Gabriels, R., Hulstaert, N., Martens, L. & Degroeve, S. DeepLC can predict retention times for peptides that carry as-yet unseen modifications. Nat. Methods 18, 1363–1369 (2021).
- Bouwmeester, R., Gabriels, R., Van Den Bossche, T., Martens, L. & Degroeve, S. The Age of Data‐Driven Proteomics: How Machine Learning Enables Novel Workflows. Proteomics 20, 1900351 (2020).
- Gabriels, R., Martens, L. & Degroeve, S. Updated MS2PIP web server delivers fast and accurate MS2 peak intensity prediction for multiple fragmentation methods, instruments and labeling techniques. Nucleic Acids Res. 47, (2019).
- C. Silva, A. S., Bouwmeester, R., Martens, L. & Degroeve, S. Accurate peptide fragmentation predictions allow data driven approaches to replace and improve upon proteomics search engine scoring functions. Bioinformatics 35, 5243–5248 (2019).